Hypothesis testing helps researchers make data-driven decisions and validate assumptions through statistical analysis. Scientists, business analysts, and healthcare professionals use this technique to determine whether sample data supports a claim about a population. By following a structured process, researchers ensure the reliability of their findings and reduce errors in decision-making. This guide walks you through the step-by-step process of hypothesis testing, provides real-life examples, and highlights common mistakes to avoid for accurate and meaningful results.
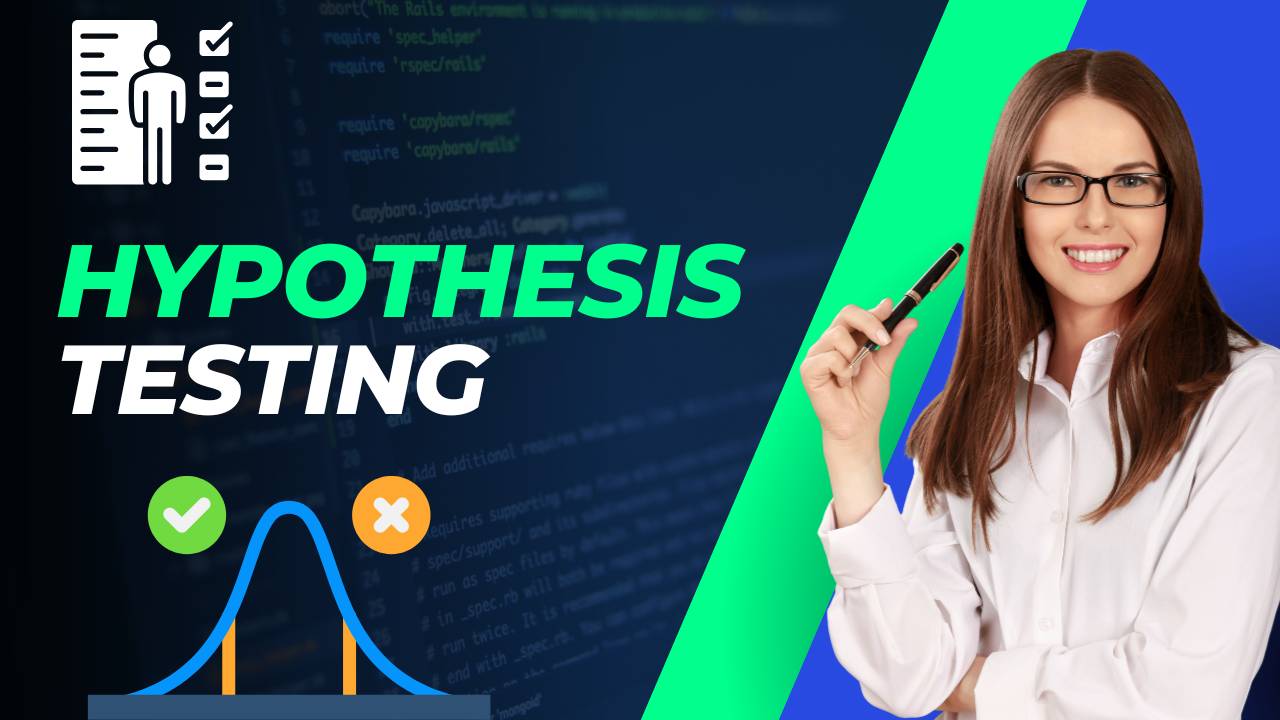
Understanding Hypothesis Testing in Research Methodology
Hypothesis testing plays an essential role in research by helping researchers evaluate claims and make data-driven decisions. It requires formulating two hypotheses: the null hypothesis (H₀), which assumes no effect or difference, and the alternative hypothesis (H₁), which suggests a significant effect or difference. Researchers collect sample data, apply statistical tests such as t-tests, chi-square tests, and ANOVA, and decide whether to reject the null hypothesis based on significance levels and p-values. Business analysts, economists, psychologists, and data scientists use hypothesis testing to validate theories, test assumptions, and improve decision-making.
To ensure accuracy, researchers carefully select statistical tests, use adequate sample sizes, and minimize errors. Type I errors (false positives) occur when they wrongly reject the null hypothesis, while Type II errors (false negatives) happen when they fail to reject a false null hypothesis. By following a structured approach, they strengthen the reliability of their findings. Hypothesis testing remains an essential tool for advancing knowledge and driving informed decisions across various fields.
Step-by-Step Hypothesis Testing Procedure for Beginners
For beginners, following a structured approach ensures accurate hypothesis testing. By understanding each step, researchers can apply statistical methods correctly and make informed decisions based on data. Below is a detailed step-by-step guide to performing hypothesis testing effectively.
1. State the Null and Alternative Hypothesis
Researchers begin by defining the two competing hypotheses. The null hypothesis (H₀) assumes that no significant effect or difference exists within the population. In contrast, the alternative hypothesis (H₁) suggests that a meaningful effect or difference does exist. Clearly stating these hypotheses helps guide the research process and determines the appropriate statistical test. For example, in a study on drug effectiveness, the null hypothesis may state that the drug has no impact, while the alternative hypothesis asserts that it improves patient outcomes.
2. Select the Significance Level (α)
Next, researchers set a significance level (α) to determine the threshold for rejecting the null hypothesis. The most commonly used significance level is 0.05 (5%), which means there is a 5% chance of rejecting a true null hypothesis. A lower significance level, such as 0.01, reduces the likelihood of errors but requires stronger evidence to reject H₀. Choosing an appropriate α level depends on the study’s field and the consequences of making an incorrect decision.
3. Choose the Appropriate Statistical Test
Researchers select the statistical test based on the type of data and research question. If they compare means between two groups, they use a t-test. If they analyze categorical data, they apply a chi-square test. When comparing means across multiple groups, they perform an ANOVA test. Selecting the right test ensures accurate results and meaningful conclusions.
4. Calculate the Test Statistic and P-Value
After choosing the test, researchers perform calculations to obtain the test statistic and p-value. The test statistic measures the degree of difference between the observed data and the null hypothesis, while the p-value quantifies the probability of obtaining the observed results if the null hypothesis is true. A small p-value (typically less than 0.05) indicates strong evidence against the null hypothesis, suggesting that the observed effect is statistically significant.
5. Draw a Conclusion
Finally, researchers interpret the p-value and make a decision. If the p-value is below the chosen significance level (α), they reject the null hypothesis (H₀) and conclude that the alternative hypothesis is likely true. If the p-value is greater than α, they fail to reject H₀, meaning the data does not provide enough evidence to support the alternative hypothesis. This conclusion guides further research, decision-making, or policy implementation based on statistical findings.
By following these structured steps, beginners can confidently apply hypothesis testing in research and make data-driven decisions with accuracy and reliability.
Types of Hypothesis Testing in Statistics: One-Tailed vs. Two-Tailed Tests
Hypothesis testing in statistics follows two main approaches: one-tailed tests and two-tailed tests. The choice between these tests depends on the research question and the expected direction of the effect. Selecting the appropriate test ensures accurate data analysis and meaningful conclusions.
1. One-Tailed Hypothesis Test
Researchers use a one-tailed test when they predict the direction of an effect before conducting the experiment. This test evaluates whether a sample statistic is significantly higher or lower than a given value, but not both. A one-tailed test provides more statistical power since it focuses on only one direction of difference.
For example, if a pharmaceutical company develops a new drug, researchers may hypothesize that the drug increases recovery speed compared to a placebo. In this case, they use a right-tailed test to check whether the drug’s effect is significantly greater than the placebo. Conversely, if they suspect that a new teaching method reduces student dropout rates, they apply a left-tailed test to determine if dropout rates decrease significantly. Since a one-tailed test does not consider the possibility of an effect in the opposite direction, researchers must justify its use before applying it.
2. Two-Tailed Hypothesis Test
A two-tailed test examines whether an effect occurs in either direction without assuming a specific outcome. Researchers use this test when they only suspect a difference but do not know whether the effect will be positive or negative. A two-tailed test splits the significance level (α) between both ends of the distribution, making it more conservative than a one-tailed test.
For example, if analysts compare the average salaries of two industries, they may hypothesize that the salaries differ but do not assume which industry pays more. In this scenario, they use a two-tailed test to check for any significant variation in either direction. Similarly, when testing the effectiveness of a new medical procedure, researchers may want to know if it performs significantly better or worse than the standard treatment, making a two-tailed test the appropriate choice.
Choosing Between One-Tailed and Two-Tailed Tests
Researchers must carefully decide whether to use a one-tailed or two-tailed test based on their hypothesis and study design. A one-tailed test increases sensitivity by focusing on a single direction but risks missing significant effects in the opposite direction.
A two-tailed test provides a more balanced approach by considering both possibilities but requires stronger evidence to achieve significance. By selecting the right test, researchers ensure the validity of their findings and improve the reliability of their statistical analysis.
Common Errors in Hypothesis Testing & How to Avoid Them
Researchers rely on hypothesis testing to make informed decisions, but several common errors can lead to incorrect conclusions. Understanding these mistakes and learning how to avoid them improves the accuracy and reliability of statistical analysis. Below are the most frequent errors in hypothesis testing and strategies to prevent them.
1. Type I Error (False Positive)
A Type I error occurs when researchers reject a true null hypothesis (H₀), mistakenly concluding that an effect exists when it does not. This error happens when the chosen significance level (α) is too high, increasing the likelihood of a false positive result. For instance, in medical testing, a Type I error could indicate that a new drug works when it actually has no effect, leading to unnecessary treatments.
To avoid Type I errors, researchers should select an appropriate significance level, commonly set at 0.05 (5%) or lower for more critical studies. Additionally, using Bonferroni corrections or other adjustments when conducting multiple comparisons helps reduce the chances of false positives.
2. Type II Error (False Negative)
A Type II error occurs when researchers fail to reject a false null hypothesis, missing a real effect. This mistake often results from insufficient sample size or low statistical power, leading to inconclusive findings. For example, if a clinical trial incorrectly concludes that a new drug has no effect when it actually improves patient outcomes, the treatment might not reach those who need it.
To minimize Type II errors, researchers should ensure their study has an adequate sample size and statistical power (typically 80% or higher). Conducting a power analysis before data collection helps determine the required sample size to detect meaningful effects.
3. Incorrect Assumptions
Every statistical test relies on specific assumptions about the data, such as normality, independence, and equal variance. If researchers fail to check these assumptions, their results may become invalid. For instance, using a t-test on data that is not normally distributed or applying an ANOVA test without checking variance equality can lead to misleading conclusions.
To avoid this error, researchers should perform assumption checks before conducting hypothesis tests. Using histograms, Q-Q plots, or Shapiro-Wilk tests helps assess normality, while Levene’s test can check for equal variances. If assumptions are violated, researchers should consider using non-parametric tests, such as the Mann-Whitney U test or Kruskal-Wallis test, which do not rely on strict assumptions.
4. Misinterpreting P-Values
Many researchers misinterpret p-values, leading to incorrect conclusions about their findings. A low p-value (typically < 0.05) suggests that the observed data is unlikely under the null hypothesis, but it does not indicate practical or real-world significance. Similarly, a high p-value does not prove that the null hypothesis is true; it only suggests insufficient evidence to reject it.
To avoid this mistake, researchers should focus on effect sizes and confidence intervals rather than relying solely on p-values. Reporting Cohen’s d, odds ratios, or confidence intervals provides a clearer picture of the study’s findings. Additionally, considering real-world implications helps ensure that statistically significant results are also meaningful in practice.
Real-Life Examples of Hypothesis Testing in Business & Clinical Trials
Hypothesis testing helps businesses optimize strategies and ensures the safety and effectiveness of medical treatments.
In business, companies use A/B testing to improve marketing, website design, and pricing strategies. For example, an e-commerce company may test a new website layout to see if it increases sales. If statistical analysis confirms a significant improvement, they implement the change to boost revenue.
In clinical trials, researchers evaluate new drugs and treatments before approval. A pharmaceutical company may test whether a new diabetes drug is more effective than the current standard. If trials show significant improvement, the drug moves forward in the approval process. Hypothesis testing also played a role in COVID-19 vaccine development by confirming their effectiveness through large-scale trials.
By applying hypothesis testing, businesses and healthcare professionals make informed, data-driven decisions that lead to better outcomes.
Hypothesis Testing vs. Confidence Intervals: Key Differences
Hypothesis testing and confidence intervals are both essential statistical methods, but they serve different purposes. It helps determine whether a claim about a population is supported by sample data, while confidence intervals provide a range of values where the true population parameter is likely to fall. The table below highlights the key differences between these two methods.
Aspect | Hypothesis Testing | Confidence Intervals |
---|---|---|
Purpose | Hypothesis testing helps determine whether there is enough statistical evidence to support or reject a claim about a population parameter. | Confidence intervals estimate a range of values within which the true population parameter is likely to fall. |
Approach | Researchers formulate a null hypothesis (H₀) and an alternative hypothesis (H₁), then use sample data to test whether H₀ should be rejected. | Researchers calculate an interval using sample data that provides a likely range for the true parameter, with a specified confidence level (e.g., 95%). |
Outcome | The test either rejects or fails to reject the null hypothesis, leading to a binary decision. | The interval provides an estimate of the parameter’s possible values, allowing for a more nuanced interpretation. |
Statistical Measure Used | Uses p-values to determine statistical significance. A p-value lower than the significance level (e.g., 0.05) indicates strong evidence against H₀. | Uses confidence levels (e.g., 90%, 95%, 99%) to indicate the probability that the true population parameter lies within the interval. |
Interpretation | A significant result suggests an effect exists, but does not indicate the size of the effect. | Provides information about the magnitude and direction of the effect, offering more context than hypothesis testing. |
Example in Business | A company tests whether a new marketing strategy increases sales compared to the current strategy by using hypothesis testing. | A company estimates that a new marketing strategy increases sales by 5% to 10%, with 95% confidence. |
Example in Healthcare | A clinical trial tests whether a new drug lowers blood pressure more effectively than an existing drug. If p < 0.05, the new drug is considered statistically significant. | A clinical trial estimates that the new drug lowers blood pressure by 8 to 12 mmHg with 95% confidence, providing an expected range of effectiveness. |
Limitations | It only gives a yes/no decision and does not indicate the size of the effect. | It does not provide a strict decision but rather an estimated range, which may lead to ambiguity. |
Both methods are valuable in statistical analysis. Hypothesis testing is useful for making clear decisions about whether an effect exists, while confidence intervals provide deeper insights into the size and range of the effect. Understanding both helps researchers and analysts make well-informed, data-driven conclusions.
Applications of Hypothesis Testing in Different Fields
Psychology & Educational Research
Hypothesis testing in psychology research helps validate theories about human behavior, while hypothesis testing in educational research evaluates the effectiveness of teaching methods.
Economics & Business
Hypothesis testing in economics is essential for analyzing market fluctuations and policy effectiveness. Companies also apply Bayesian hypothesis testing explained as an alternative approach for making probabilistic inferences.
Machine Learning & Quality Control
Hypothesis testing in machine learning ensures models perform as expected, while hypothesis testing in quality control helps maintain manufacturing standards.
Performing Hypothesis Testing Using Statistical Software
Advancements in statistical software have made hypothesis testing more accessible and efficient across various fields. Researchers, analysts, and data scientists now rely on tools like Excel, R, and Python to perform statistical tests with greater accuracy and speed. These tools help automate complex calculations, visualize data, and interpret results effectively.
Hypothesis Testing Using Excel
Excel provides built-in functions and the Analysis ToolPak to perform basic hypothesis tests, including t-tests, ANOVA, and regression analysis. Users can input their dataset, select the appropriate test, and generate outputs such as p-values, test statistics, and confidence intervals.
For example, in a two-sample t-test, Excel allows users to compare two population means and determine whether a significant difference exists. The software simplifies hypothesis testing for professionals in business, finance, and healthcare who need quick statistical insights without extensive programming knowledge.
Hypothesis Testing Using R Programming
R is a powerful statistical programming language that researchers and data scientists use for advanced statistical modeling and hypothesis testing. R provides built-in functions such as t.test()
, chisq.test()
, and anova()
to perform t-tests, chi-square tests, and ANOVA efficiently.
The ggplot2 and dplyr packages enhance data visualization and manipulation, making hypothesis testing more comprehensive. For example, in clinical trials, researchers use R to test the effectiveness of new treatments by comparing different patient groups and visualizing results with boxplots and histograms.
Hypothesis Testing Using Python
Python has become a dominant tool in data science and machine learning, with libraries like SciPy, statsmodels, and pandas enabling sophisticated hypothesis testing. The scipy.stats
module includes functions for t-tests, chi-square tests, and Wilcoxon tests, helping analysts conduct rigorous statistical analyses.
Python’s ability to handle large datasets and automate repetitive tasks makes it ideal for predictive analytics and A/B testing in marketing and finance. For example, an e-commerce company can use Python to test whether a new website design leads to higher sales conversions by analyzing user behavior data.
Effect Size, Power Analysis & Limitations of Hypothesis Testing
Hypothesis testing plays a important role in statistical analysis, but understanding its effectiveness requires more than just statistical significance. Researchers use effect size and power analysis to assess the practical significance and reliability of their findings. Limitations of hypothesis testing can impact the accuracy and interpretation of results.
Effect Size in Hypothesis Testing
Effect size quantifies the strength or magnitude of the relationship between variables, providing context beyond just statistical significance. A small p-value may indicate statistical significance, but it does not reveal whether the effect is meaningful in real-world applications. Common measures of effect size include Cohen’s d (for mean differences), Pearson’s r (for correlation), and eta squared (η²) (for variance explained in ANOVA). Researchers in fields like psychology, medicine, and economics use effect size to determine whether an observed change is practically significant rather than just statistically significant.
For example, in a clinical drug trial, a new medication may show a statistically significant reduction in symptoms compared to a placebo. If the effect size is small, the improvement may not be clinically meaningful for patients. Therefore, reporting effect size along with hypothesis test results helps make better decisions about the importance of findings.
Power Analysis in Hypothesis Testing
Power analysis determines the likelihood of detecting a true effect when one exists. The statistical power of a test depends on four key factors:
- Sample Size – A larger sample increases the ability to detect a true effect.
- Effect Size – A stronger effect is easier to detect with a smaller sample.
- Significance Level (α) – Lower α (e.g., 0.01 instead of 0.05) reduces Type I errors but requires a larger sample for sufficient power.
- Variability in Data – Higher variability reduces power, making it harder to detect true differences.
Researchers typically aim for 80% power (0.80), meaning they have an 80% probability of correctly rejecting the null hypothesis if an effect exists. In business decision-making, power analysis in A/B testing helps determine how many customers need to be tested to detect a meaningful difference in conversion rates.
Limitations of Hypothesis Testing
While hypothesis testing is a valuable tool, it has several limitations that researchers must consider:
- Dependence on Sample Data – Hypothesis tests rely on sample data, which may not accurately represent the entire population. If the sample is biased or too small, the results may be misleading.
- Misinterpretation of P-Values – Many assume a low p-value confirms a strong effect, but statistical significance does not guarantee practical significance.
- Influence of Sample Size – With large samples, even tiny differences can become statistically significant, leading to misleading conclusions about real-world relevance.
- Assumption Violations – Many hypothesis tests assume normal distribution, equal variances, or independent observations. Violating these assumptions can distort results.
- Potential for Type I and Type II Errors – Researchers risk making incorrect conclusions by rejecting a true null hypothesis (Type I error) or failing to reject a false null hypothesis (Type II error).
Common Misconceptions About Hypothesis Testing
Hypothesis testing is a fundamental statistical method, but many people misinterpret its results, leading to incorrect conclusions. Common misconceptions often arise from a lack of understanding of statistical significance, over-reliance on p-values, and misinterpretation of null hypothesis rejection. To draw accurate and meaningful conclusions, researchers must go beyond hypothesis testing alone and incorporate complementary statistical tools.
Misconception 1: Statistical Significance Implies Practical Importance
One of the most common misunderstandings in hypothesis testing is equating statistical significance with real-world relevance. A small p-value (e.g., p < 0.05) only indicates that the observed effect is unlikely due to random chance, not that the effect is large or meaningful.
For example, in a clinical trial, a new drug may show a statistically significant reduction in blood pressure. If the average reduction is only 0.5 mmHg, the effect might be too small to provide any real health benefit to patients. Researchers should always analyze effect size alongside statistical significance to determine whether an effect has practical value.
Misconception 2: A Non-Significant Result Means No Effect Exists
Another misconception is that failing to reject the null hypothesis (H₀) means the effect does not exist. In reality, a non-significant p-value only suggests that the study lacks enough evidence to conclude an effect is present—it does not prove the null hypothesis is true.
For instance, in business decision-making, a company may test a new marketing strategy against an existing one. If the test fails to show significance, it does not mean the new strategy is ineffective. It could be due to small sample size, high variability, or insufficient statistical power. Researchers should conduct power analysis and consider confidence intervals to better interpret results.
Misconception 3: P-Value Confirms the Null Hypothesis is False
A p-value below 0.05 does not prove the alternative hypothesis is true; it only suggests that the data provides enough evidence to reject the null hypothesis. Many assume that a significant result means a finding is definitive, but statistical results always involve a degree of uncertainty.
For example, in psychological research, a study might find that a new therapy improves anxiety symptoms with a p-value of 0.03. This result suggests some evidence against the null hypothesis, but it does not confirm with absolute certainty that the therapy works for all individuals. Replication studies and confidence intervals provide stronger support for conclusions.
Misconception 4: Single Study Provides Definitive Proof
Relying on a single hypothesis test to make broad conclusions is a major mistake. Scientific research requires replication, meaning multiple studies must produce consistent results before a conclusion is widely accepted.
For example, in medical research, a single experiment may show that a certain diet improves heart health, but unless other independent studies replicate the results, the conclusion remains uncertain. Meta-analysis and systematic reviews help assess the reliability of findings by aggregating results from multiple studies.
Misconception 5: P-Values Can Measure the Probability of a Hypothesis Being True
Many believe that a p-value of 0.02 means there is only a 2% chance the null hypothesis is true, but this is incorrect. A p-value only tells us how likely the observed data would occur if the null hypothesis were true—it does not provide the probability that H₀ or H₁ is correct.
For example, in financial market research, a statistical test might suggest that a stock-picking strategy outperforms the market with a p-value of 0.01. This does not mean the strategy will work 99% of the time—it only means that if the market were random, the observed outperformance would be unlikely. Bayesian statistics provide better ways to quantify the probability of hypotheses.
Overcoming Misconceptions with Complementary Statistical Methods
To avoid misinterpretations, researchers should use multiple statistical tools alongside hypothesis testing:
- Confidence Intervals – Provide a range of likely values for an effect size instead of a binary decision from a p-value.
- Effect Size Metrics – Quantify the practical significance of findings, helping to determine if results are meaningful.
- Power Analysis – Ensures that a study has sufficient sample size to detect real effects.
- Replication Studies – Confirm findings by testing the same hypothesis across different datasets and populations.
FAQs
1. What is the role of sample size in hypothesis testing?
A larger sample size increases statistical power, reduces variability, and leads to more reliable results, making it easier to detect real effects.
2. What are effect sizes, and why are they important?
Effect size quantifies the strength of a relationship or difference between groups, helping researchers determine if a statistically significant result is also practically meaningful.
3. Can hypothesis testing be used in machine learning?
Yes, hypothesis testing is widely used in machine learning to evaluate model performance, compare algorithms, and validate assumptions.
4. How does hypothesis testing apply in business?
Businesses use hypothesis testing in A/B testing, pricing strategies, customer behavior analysis, and quality control to make data-driven decisions.
5. What is the difference between hypothesis testing and confidence intervals?
- Hypothesis testing determines if an effect exists.
- Confidence intervals estimate the range in which the true effect likely falls.
6. Can a hypothesis test prove a hypothesis to be true?
No, hypothesis testing only provides evidence to support or reject a hypothesis—it does not prove it with certainty.
7. What are some common mistakes in hypothesis testing?
- Misinterpreting p-values as proof of truth.
- Ignoring effect size and confidence intervals.
- Conducting multiple tests without adjusting for errors.
8. What is the difference between parametric and non-parametric tests?
- Parametric tests assume data follows a normal distribution (e.g., t-test, ANOVA).
- Non-parametric tests do not assume normality (e.g., Mann-Whitney U test, Kruskal-Wallis test).
9. How is hypothesis testing used in clinical trials?
Researchers use hypothesis testing to determine if new treatments or drugs are significantly better than existing ones before approval.
10. What statistical software is commonly used for hypothesis testing?
- Excel – For basic t-tests and regression analysis.
- R – For advanced statistical modeling.
- Python – For data science applications and predictive analytics.