In today’s data-driven world, businesses rely on data analysis methods to extract meaningful insights, enhance efficiency, and drive strategic decisions. From predictive analytics to machine learning algorithms, modern enterprises are leveraging advanced techniques to stay ahead of the competition.
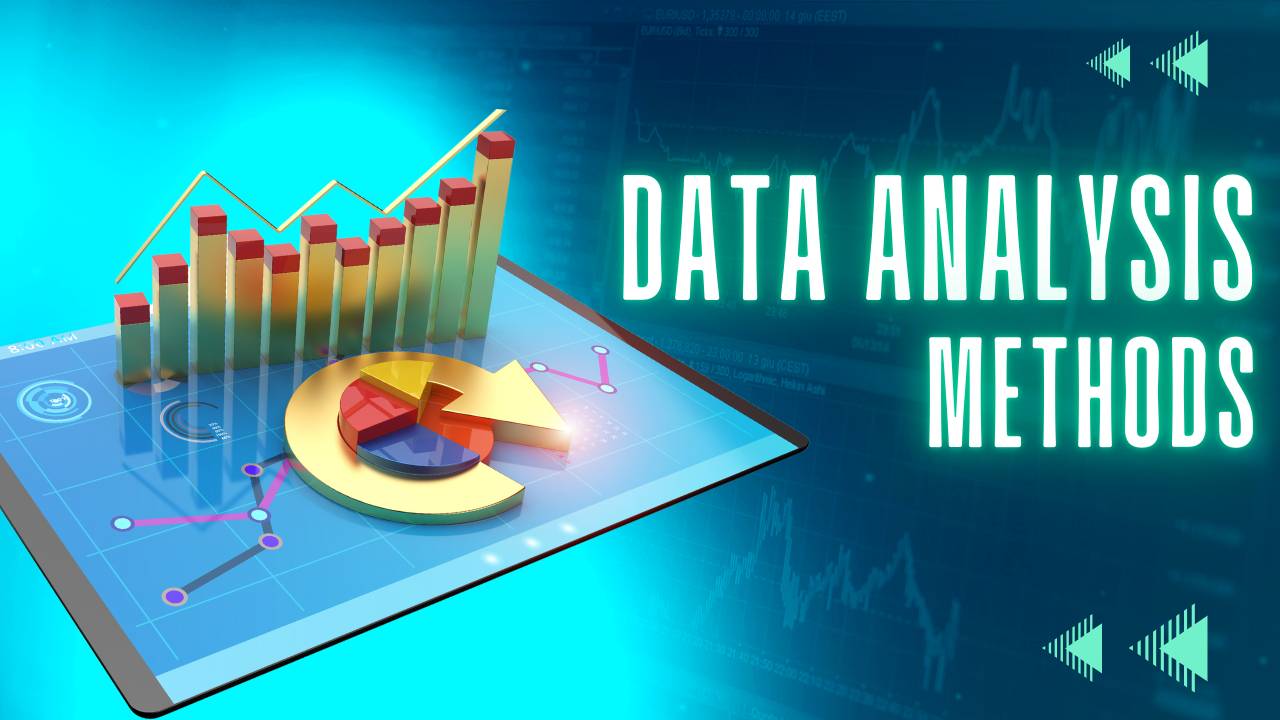
As industries evolve, the demand for robust data analysis methods continues to grow, shaping the future of decision-making in business. By integrating cutting-edge analytical tools, organizations can unlock hidden patterns, optimize operations, and make data-backed decisions with confidence.
Understanding Data Analysis Methods
Data analysis methods encompass a wide range of techniques used to collect, process, and interpret raw data, transforming it into valuable insights that drive informed decision-making. These methods help businesses uncover patterns, detect anomalies, and predict future trends, enabling them to optimize strategies and improve overall efficiency.
In today’s competitive landscape, companies rely on data analysis methods to enhance customer experiences, streamline operations, and maximize profitability. Whether it’s identifying market trends, analyzing consumer behavior, or forecasting demand, these techniques play a essential role in shaping business success.
Choice of data analysis methods varies based on factors such as data type (qualitative or quantitative), industry requirements, and the complexity of the insights needed. Some businesses utilize traditional statistical approaches, while others integrate advanced machine learning and artificial intelligence for real-time analytics. As technology evolves, these methods continue to adapt, making data-driven decision-making more precise and efficient than ever before.
Types of Data Analysis Methods
Data analysis methods provide businesses with powerful tools to extract insights, identify trends, and make data-driven decisions. Depending on the purpose and complexity of the analysis, different techniques are used to process and interpret data effectively.
Below are the key types of data analysis methods, along with detailed explanations of their significance, applications, and real-world examples.
- Descriptive Analysis
- Diagnostic Analysis
- Predictive Analysis
- Prescriptive Analysis
- Exploratory Data Analysis (EDA)
- Inferential Analysis
- Text Analysis (Natural Language Processing – NLP)
1. Descriptive Analysis
Descriptive analysis is the foundation of data analysis, focusing on summarizing past data to identify patterns, trends, and key insights. It provides a historical view of business performance and helps organizations understand what has already happened.
Applications:
- Used in financial reporting to assess company revenue, profit, and expenses over time.
- Helps businesses analyze sales performance and customer demographics.
- Enables marketing teams to evaluate the success of past campaigns.
Example: A retail company reviews last year’s sales data to identify peak shopping seasons. By examining monthly revenue trends, the company can determine which months saw the highest sales and adjust marketing efforts accordingly.
2. Diagnostic Analysis
Diagnostic analysis goes beyond descriptive analysis by explaining why certain trends or patterns occurred. It involves in-depth data exploration to uncover causes behind changes in key business metrics.
Applications:
- Identifies reasons for fluctuations in customer retention, revenue, or operational efficiency.
- Helps businesses understand the impact of external factors (e.g., economic shifts, competitor actions) on performance.
- Used in healthcare to determine the cause of disease outbreaks.
Example: An e-commerce company notices a sudden drop in customer retention rates. Through diagnostic analysis, the company finds that increased competition and slower delivery times are the primary reasons behind the decline.
3. Predictive Analysis
Predictive analysis uses historical data, statistical algorithms, and machine learning to forecast future outcomes. It helps businesses anticipate potential risks, customer behaviors, and market trends.
Applications:
- Used by financial institutions to predict stock market trends and assess credit risks.
- Helps retailers forecast customer demand during different seasons.
- Enables insurance companies to estimate claim probabilities.
Example: A retail company analyzes past holiday shopping trends and combines them with current market conditions to predict which products will be in high demand. This allows the company to stock up on inventory before the shopping season begins.
4. Prescriptive Analysis
Prescriptive analysis takes predictive analysis a step further by recommending specific actions based on data-driven forecasts. It leverages AI, machine learning, and optimization techniques to suggest the best course of action for a given situation.
Applications:
- Used in supply chain management to optimize logistics and delivery routes.
- Helps healthcare providers determine personalized treatment plans based on patient data.
- Assists businesses in making data-backed pricing and marketing decisions.
Example: A logistics company integrates AI-powered analytics to optimize delivery routes in real-time. By analyzing traffic patterns, weather conditions, and customer locations, the system suggests the fastest and most efficient routes for deliveries.
5. Exploratory Data Analysis (EDA)
Exploratory Data Analysis (EDA) is used to identify hidden patterns, relationships, and anomalies within datasets. Unlike traditional analysis, EDA does not start with predefined hypotheses but instead helps analysts discover new insights by visualizing and summarizing raw data.
Applications:
- Used in research to explore datasets before forming hypotheses.
- Helps businesses detect unusual patterns, such as fraud or unexpected consumer behavior.
- Common in healthcare analytics to uncover new disease risk factors.
Example: A healthcare company collects patient data and uses EDA to identify potential correlations between lifestyle factors and the risk of heart disease. This analysis helps in designing better preventive care strategies.
6. Inferential Analysis
Inferential analysis allows analysts to draw conclusions about an entire population based on a sample dataset. This statistical method is widely used in scientific research, business forecasting, and social studies.
Applications:
- Used in market research to estimate consumer preferences based on survey samples.
- Helps pharmaceutical companies test the effectiveness of new drugs before mass production.
- Assists economists in predicting GDP growth based on limited economic indicators.
Example: A pharmaceutical company conducts a clinical trial with 1,000 patients to test the effectiveness of a new drug. If the results show a positive outcome, the findings are applied to a larger population before regulatory approval.
7. Text Analysis (Natural Language Processing – NLP)
Text analysis, often powered by Natural Language Processing (NLP), involves analyzing textual data from various sources to extract meaningful insights. It is commonly used in sentiment analysis, content classification, and automated customer interactions.
Applications:
- Used in social media monitoring to analyze customer sentiment about brands.
- Helps businesses understand customer reviews and feedback.
- Enables chatbots and virtual assistants to process and respond to human language effectively.
Example: A social media monitoring tool analyzes thousands of customer comments about a new product launch. By categorizing comments as positive, neutral, or negative, businesses can gauge public perception and make data-driven improvements.
Role of Data Analysis in Business Decision-Making
Data analysis has become an integral part of modern business operations, driving data-driven decision-making across various industries. Businesses leverage different data analysis methods to optimize strategies, improve customer satisfaction, enhance operational efficiency, and mitigate risks.
Below is a detailed breakdown of how data analysis methods contribute to business decision-making and long-term success.
Enhancing Strategic Planning
Businesses can make informed strategic decisions by analyzing historical and real-time data. By identifying key performance indicators (KPIs) and market trends, companies can refine their growth strategies and minimize risks.
Improving Customer Experience
With customer behavior analysis, businesses can tailor their services and products to meet specific demands. Personalization, recommendation engines, and AI-driven customer support enhance user satisfaction and brand loyalty.
Optimizing Operations and Efficiency
Data analysis helps in resource allocation, supply chain management, and process automation. Companies can reduce costs, improve productivity, and streamline workflows by utilizing predictive and prescriptive analytics.
Risk Management and Fraud Detection
Data-driven insights enable companies to assess risks, detect fraud, and prevent financial losses. Advanced analytics methods, including machine learning, identify suspicious activities in banking, insurance, and e-commerce sectors.
Emerging Trends in Data Analysis Methods
As businesses become increasingly data-driven, data analysis methods are evolving to keep up with the rising complexity and volume of information. New technologies and methodologies are shaping the future of decision-making by enhancing accuracy, speed, and accessibility.
Below, are key emerging trends that are transforming how businesses analyze and interpret data for strategic decision-making.
Artificial Intelligence and Machine Learning
AI-powered data analysis methods are revolutionizing business decision-making. Machine learning algorithms continuously improve predictive accuracy, enabling businesses to adapt to dynamic market conditions.
Big Data Analytics
With the exponential growth of data, businesses are leveraging big data analytics to handle massive datasets. Technologies like Hadoop and Spark process large volumes of data in real-time, providing deeper insights and faster decision-making.
Real-Time Data Processing
Businesses no longer rely solely on historical data; real-time data processing allows instant analysis and decision-making. Industries such as finance, healthcare, and e-commerce benefit from real-time customer interactions and fraud detection.
Cloud-Based Analytics
Cloud computing facilitates scalable and cost-effective data analysis solutions. Businesses can store and analyze vast amounts of data without heavy infrastructure investments, making analytics accessible to enterprises of all sizes.
Blockchain for Data Integrity
Blockchain technology ensures secure and tamper-proof data storage, enhancing data reliability and trust in financial transactions, supply chains, and digital identity management.
Automated Data Analysis Tools
User-friendly automated tools like Tableau, Power BI, and Google Data Studio make data analysis methods accessible to non-technical users, enabling self-service analytics for better decision-making.
Challenges in Implementing Data Analysis Methods
While data analysis methods offer significant advantages for businesses, implementing them effectively comes with several challenges. Companies must address issues related to security, data integrity, talent shortages, and technological constraints to fully leverage the power of analytics.
Below are some of the most pressing challenges that organizations face in data analysis and strategies to overcome them.
1. Data Privacy and Security
As businesses collect and analyze vast amounts of data, privacy and security have become major concerns. The risk of data breaches, cyberattacks, and unauthorized access to sensitive information has increased, leading to stringent data protection laws.
Key Challenges:
- Businesses must comply with global regulations such as the General Data Protection Regulation (GDPR) in Europe and the California Consumer Privacy Act (CCPA) in the U.S., which impose strict guidelines on data collection, storage, and processing.
- Cybersecurity threats such as phishing attacks, ransomware, and insider threats pose risks to confidential business and customer data.
- Many companies struggle with data encryption and access control, leading to vulnerabilities in their data protection strategies.
Potential Solutions:
- Implementing strong data encryption, multi-factor authentication (MFA), and access control mechanisms can safeguard sensitive data.
- Businesses should regularly conduct security audits to identify vulnerabilities and address potential threats before they cause damage.
- Using privacy-preserving technologies like differential privacy and federated learning can allow businesses to analyze data while minimizing exposure to personal information.
Example: A financial institution handling sensitive customer transactions must ensure compliance with GDPR by anonymizing personal data before conducting analytics. Implementing AI-driven fraud detection systems can also prevent data breaches.
2. Data Quality and Integration
Poor data quality and lack of integration across different systems hinder the effectiveness of data analysis methods. If data is inconsistent, incomplete, or duplicated across various departments, businesses may derive misleading insights that result in flawed decision-making.
Key Challenges:
- Organizations often deal with disparate data sources, including CRM systems, IoT devices, social media, and enterprise applications, leading to data silos that prevent seamless integration.
- Inaccurate or outdated data can lead to misleading analytics results, affecting business strategies and operations.
- Data cleaning and preprocessing require significant time and resources, especially in large-scale data environments.
Potential Solutions:
- Companies should implement Master Data Management (MDM) strategies to unify data across departments and eliminate redundancies.
- Automated data cleaning tools powered by AI can help detect inconsistencies, correct errors, and standardize datasets.
- Cloud-based data lakes and data warehouses provide centralized repositories where structured and unstructured data can be stored, processed, and analyzed efficiently.
Example: A multinational retailer operating across multiple regions integrates data from its online and offline stores into a centralized data warehouse. This ensures real-time access to clean, unified data for sales forecasting and inventory management.
3. Skill Gaps in Data Science
One of the most significant challenges businesses face in implementing data analysis methods is the shortage of skilled professionals in data science, analytics, and machine learning. The demand for data scientists, analysts, and AI specialists has surged, but there are not enough qualified professionals to fill these roles.
Key Challenges:
- Businesses struggle to find employees with expertise in statistical modeling, machine learning, and data visualization.
- The complexity of data analysis tools and programming languages like Python, R, and SQL creates barriers for non-technical employees.
- Many organizations rely on traditional reporting methods instead of advanced analytics due to a lack of in-house expertise.
Potential Solutions:
- Investing in employee training programs and partnerships with universities can help bridge the skill gap and cultivate a data-driven workforce.
- Implementing self-service analytics tools like Tableau, Power BI, and Google Data Studio allows non-technical users to generate insights without relying on data science teams.
- Businesses can leverage AI-powered automated analytics platforms that require minimal coding expertise, making data analysis more accessible across all departments.
Example: A manufacturing company lacking in-house data scientists integrates AI-driven business intelligence tools, enabling managers to analyze production efficiency using intuitive dashboards without coding expertise.
4. High Implementation Costs and Infrastructure Challenges
Adopting advanced data analysis methods requires a significant investment in infrastructure, software, and human resources. Businesses, especially small and medium enterprises (SMEs), often struggle with the costs associated with data analytics implementation.
Key Challenges:
- Setting up data storage solutions, such as on-premise servers or cloud-based platforms, can be expensive.
- Licensing costs for business intelligence (BI) tools and machine learning platforms can be prohibitive for smaller organizations.
- Scaling analytics solutions as businesses grow requires continuous infrastructure upgrades, which may strain budgets.
Potential Solutions:
- Cloud-based analytics services (such as AWS, Google Cloud, and Azure) offer scalable and cost-effective solutions, allowing businesses to pay for only what they use.
- Open-source tools like Apache Hadoop, TensorFlow, and KNIME provide cost-efficient alternatives to expensive enterprise solutions.
- Companies can start with small-scale pilot projects to assess the impact of data analytics before making full-scale investments.
Example: A startup in the e-commerce sector uses Google Cloud BigQuery to analyze customer purchase trends, avoiding the high costs of setting up an on-premise data warehouse.
5. Ethical and Bias Issues in Data Analysis
The ethical implications of data analysis are becoming increasingly important as businesses use AI-driven analytics for decision-making. Unchecked biases in data collection, algorithm design, and model training can lead to unfair or discriminatory outcomes.
Key Challenges:
- AI models trained on biased data can reinforce discrimination in hiring, lending, and law enforcement decisions.
- Lack of transparency in AI-driven analytics can make it difficult to explain decisions and ensure fairness.
- Companies must navigate complex ethical issues related to data ownership, consent, and algorithmic accountability.
Potential Solutions:
- Businesses should implement bias detection and mitigation strategies to ensure fair and ethical use of data.
- Using explainable AI (XAI) techniques can provide insights into how machine learning models generate predictions, improving transparency and accountability.
- Ethical AI frameworks and compliance guidelines should be integrated into corporate governance policies.
Example: A financial institution reviews its AI-powered credit approval system to detect potential biases in lending decisions and ensures fair access to loans for all customer demographics.
Future of Business Decision-Making with Data Analysis
As businesses adopt AI, big data, and real-time analytics, decision-making will become more automated, precise, and insight-driven. Companies that leverage these advanced data analysis methods will gain a strong competitive advantage, enabling them to adapt quickly to market changes, improve efficiency, and enhance customer experiences.
1. Hyper-Personalization
AI-powered insights will allow businesses to analyze individual customer behavior, preferences, and past interactions to deliver tailored products and services. Retail, finance, healthcare, and entertainment platforms will use machine learning algorithms to recommend personalized solutions, boosting customer satisfaction and engagement.
2. Autonomous Decision-Making
With AI and machine learning, organizations will shift towards real-time automated decision-making, reducing reliance on human intervention. This will optimize supply chains, automate financial transactions, enhance fraud detection, and improve predictive maintenance in industries like manufacturing and healthcare.
3. Augmented Analytics
AI-driven analytics tools will provide businesses with advanced data visualization, automated insights, and predictive recommendations, making data more accessible to decision-makers. Tools like Google Data Studio, Tableau, and Power BI will simplify complex datasets, allowing businesses to make strategic decisions faster and more accurately.
4. Real-Time Decision-Making with Edge AI
Edge computing will enable businesses to process data instantly at its source, reducing latency and improving efficiency. This technology will be essential for industries such as smart cities, autonomous vehicles, IoT-driven logistics, and real-time financial trading, where split-second decisions are necessary.
5. AI-Driven Risk Management
Future decision-making will rely heavily on AI-powered risk analysis, helping businesses mitigate potential threats before they occur. Predictive analytics and anomaly detection models will allow financial institutions, cybersecurity firms, and healthcare organizations to identify fraud, security breaches, and operational risks in real-time.
6. Data-Driven Corporate Strategies
Companies will increasingly use big data and AI insights to shape long-term strategies, from market expansion and product development to workforce planning and sustainability initiatives. Businesses that harness real-time analytics, consumer behavior insights, and competitive intelligence will stay ahead in an increasingly data-centric world.
FAQs
1. How does data analysis improve customer experience?
By analyzing customer behavior and feedback, businesses can personalize recommendations, enhance services, and predict customer needs, leading to higher satisfaction and retention.
2. What is exploratory data analysis (EDA)?
EDA is an initial approach to examining datasets, identifying patterns, and uncovering relationships before performing formal modeling or hypothesis testing.
3. How does blockchain enhance data analysis?
Blockchain ensures data integrity, transparency, and security by preventing tampering, making it valuable in financial transactions, supply chains, and digital identity management.
4. What are the best tools for data analysis?
Popular tools include Python, R, SQL, Tableau, Power BI, Google Data Studio, SAS, and Hadoop for processing, visualizing, and interpreting data.
5. What is text analysis, and how is it used in business?
Text analysis, or Natural Language Processing (NLP), helps businesses extract insights from customer reviews, social media sentiment, emails, and chatbot interactions.
6. How does data analysis contribute to risk management?
Businesses use predictive analytics and anomaly detection to identify and mitigate potential risks, such as fraud, cybersecurity threats, and financial losses.
7. What is inferential analysis?
Inferential analysis allows businesses to make conclusions about a larger population based on a sample dataset, often used in surveys and market research.
8. How does data-driven decision-making impact business growth?
By using real-time insights, businesses can reduce costs, improve efficiency, enhance customer engagement, and create innovative products, leading to long-term growth.
9. What is the future of data analysis in business?
The future will see AI-driven analytics, real-time data processing, hyper-personalization, autonomous decision-making, and edge computing becoming mainstream in business operations.
10. How can small businesses benefit from data analysis?
Even small businesses can use affordable data analytics tools to track customer preferences, optimize marketing efforts, manage inventory, and improve operational efficiency.